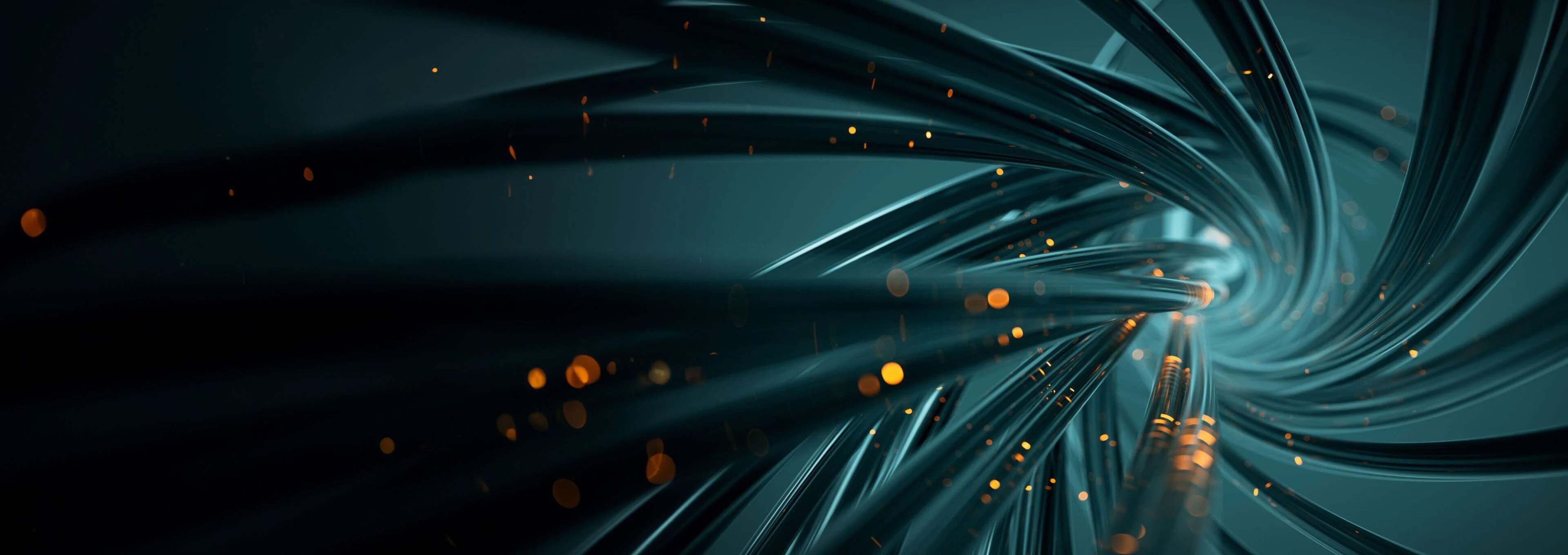
MediaTek
人工智慧
引領邊緣人工智慧技術革命
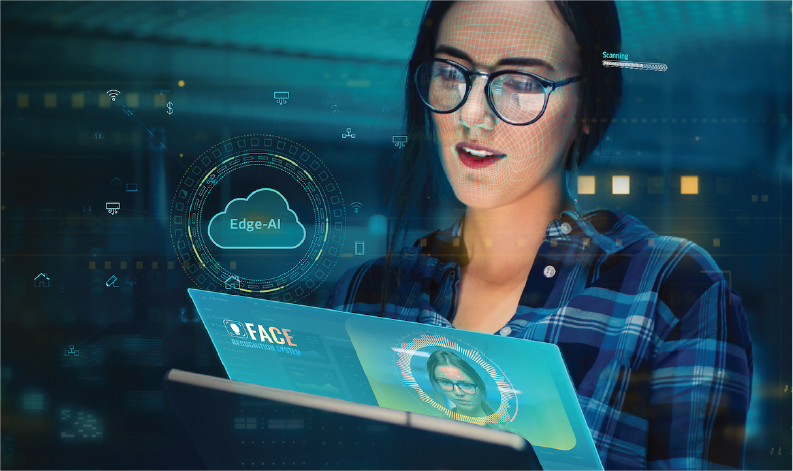
邊緣人工智慧
人工智慧增強用例的快速發展正在改變設備的創建和使用方式。這種需求的轉變以及人工智慧技術能力的不斷增強,正在促使產品在家庭、車輛或個人產品的「邊緣」處理人工智慧增強功能,而不是僅僅依賴雲端連接的支援。
這種邊緣運算的優點包括即時性、資料隱私性和整體功耗較低。對於設備製造商而言,也無需在產品銷售的每個地區推出支援雲端基礎設施,從而縮短了上市時間。
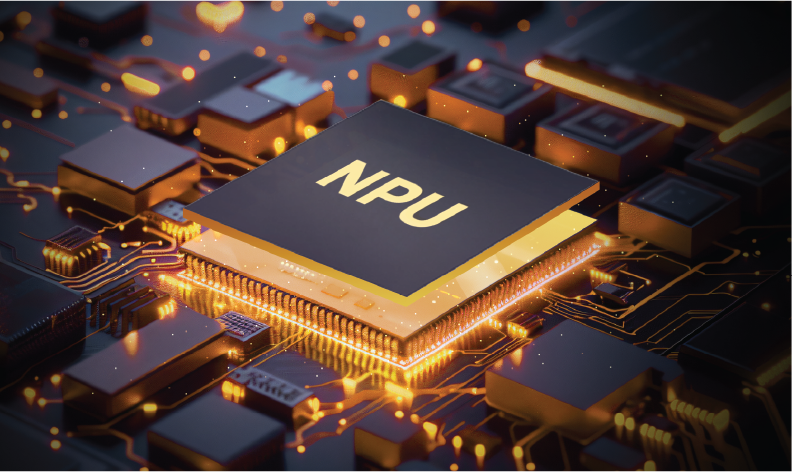
MediaTek 神經處理單元 (NPU)
MediaTek 開發自己的深度學習加速器(性能核心)、視覺處理單元(靈活核心)、基於硬體的多核心調度器和軟體開發套件(NeuroPilot),構成其業界領先的神經處理單元(NPU)的核心組件。
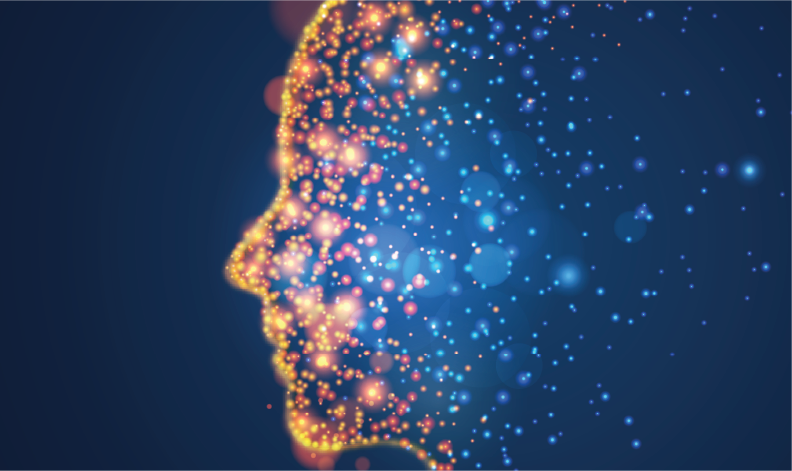
MediaTek 神經飛行員
我們正在透過 MediaTek NeuroPilot 正面應對 Edge AI 挑戰。透過 APU、GPU 和 CPU 等 SoC 中的異質運算功能,我們為 AI 功能和應用程式提供高效能和高能源效率。開發人員可以針對晶片內的這些特定處理單元,或讓 MediaTek NeuroPilot SDK 智慧地處理它們的處理分配。
MediaTek 生成式人工智慧
作為開發功能強大、高度整合和高效系統系統產品的產業領導者,MediaTek 透過創建邊緣人工智慧硬體處理生態系統,並與其產品系列(智慧型手機和筆記型電腦、智慧家庭、企業智慧物聯網和智慧汽車)中的綜合軟體工具相結合,實現人工智慧的未來。
今天、明天與未來
現在
隨著人工智慧的快速發展,它正在重塑我們在家裡,工作場所和城市中所使用的技術,並為我們帶來新的體驗以及改變我們的互動方式。
現在,人工智慧能夠實現如深度學習面部檢測(DL-FD)、即時美化、創意圖層堆疊、物體和場景辨識、AR/MR 加速、攝影和視頻的增強現實等技術。
明天及未來
先進人工智慧裝置的未來是巨大的。想像一下,未來可使用完全符合您需求和習慣的裝置:透過智慧型手機追蹤您的健康狀況,並在您身體感到任何不適前就預先提醒您注意;建造智慧家庭的環境,可以在您到家之前就先開啟家中的電燈和暖氣;或乘坐自動駕駛汽車載您到任何您想去的地方。這種與生俱來的智慧如此貼近我們的生活,帶來了新的用戶體驗水準,並改變了您的世界。這就是終端人工智慧落地的體現。
MediaTek NeuroPilot 特點
一次編寫, 隨處應用
聯發科技 NeuroPilot SDK 支援所有聯發科技具人工智慧的硬體。 它允許開發人員為現有和未來的聯發科技硬體平台以及包括 智慧手機,汽車,智慧家庭,物聯網等在內的所有產品線做“一次編寫,隨處應用”。 這不僅簡化創建過程,也節省成本和上市時間。 其所支援的軟體生態系統包括安卓和 Linux 作業系統, 並提供完整的編譯器,分析器和應用程式庫。
構建友善的架構
開發者可以使用 TensorFlow、TF Lite、Caffe、Caffe2 Amazon MXNet、Sony NNabla 或其他自訂的協力廠商通用架構來構建應用程式。在 API 級別,我們提供聯發科技 NeuroPilot SDK 包括谷歌安卓神經網路 API(Android NNAPI)和聯發科技 NeuroPilot 擴充元件,從而讓開發人員和設備製造商能以更加貼近硬體的方式編碼以提高性能和省電效率。
MediaTek 研究
聯發科技研究院致力於發展和提升日常設備中的人工智慧生態系統。
這包括具有極高解析度的感測器、專門用於散景捕捉等流行功能的感測器、用於增強光靈敏度的單聲道感測器或用於獨特應用的專業感測器。隨附的鏡頭可以適應多種拍攝風格,包括變焦、廣角、微距或日常攝影。
Mar 8, 2023
Extending the Pre-Training of BLOOM for Improved Support of Traditional Chinese: Models, Methods and Results
In this paper we present the multilingual language model BLOOM-zh that features enhanced support for Traditional Chinese. BLOOM-zh has its origins in the open-source BLOOM models presented by BigScience in 2022.
Feb 02, 2023
Fisher-Legendre (FishLeg) optimization of deep neural networks
We introduce a new approach to estimate the natural gradient via Legendre-Fenchel duality, provide a convergence proof, and show competitive performance on a number of benchmarks.
Dec 19, 2022
A Learning-Based Algorithm for Early Floorplan With Flexible Blocks
This paper presents a learning-based algorithm using graph neural network (GNN) and deconvolution network to predict the placement of the locations and the aspect ratios for the design blocks with flexible rectangles.
Oct 31, 2022
Near-Optimal Collaborative Learning in Bandits
A near-optimal algorithm is proposed for pure exploration in a new framework for collaborative bandit learning that encompasses recent prior works.
Nov 24, 2022
Gradient Descent: Robustness to Adversarial Corruption
We provide performance guarantees for gradient descent under a general adversarial framework
Improved Convergence Rates for Sparse Approximation Methods in Kernel-Based Learning
Kernel-based models such as kernel ridge regression and Gaussian processes are ubiquitous in machine learning applications for regression and optimization.
Feb 20, 2022
Regret Bounds for Noise-Free Kernel-Based Bandits
Kernel-based bandit is an extensively studied black-box optimization problem, in which the objective function is assumed to live in a known reproducing kernel Hilbert space.
LPI: Learned Positional Invariances for Transfer of Task Structure and Zero-shot Planning
Real-world tasks often include interactions with the environment where our actions can drastically change the available or desirable long-term outcomes.
Jun 1, 2022
Adaptive erasure of spurious sequences in sensory cortical circuits
Sequential activity reflecting previously experienced temporal sequences is considered a hallmark of learning across cortical areas.
Apr 13, 2022
Flexible Multiple-Objective Reinforcement Learning for Chip Placement
Recently, successful applications of reinforcement learning to chip placement have emerged. Pretrained models are necessary to improve efficiency and effectiveness.